In recent years, artificial intelligence (AI) has become an indispensable tool for businesses across different enterprises, including the financial industry. According to the World Economic Forum survey in 2020, around 85 percent of financial institutions have begun incorporating some form of AI technology into their systems. AI is most notably beneficial for significantly enhancing anti money laundering (AML) systems in banks to efficiently monitor clients and accurately detect illicit financial transactions.
AI can interpret information in ways that emulate how humans think and organize data. Specifically, AI that uses machine learning is capable of improving how it performs various tasks without direct programming. It does this by interacting with humans and constantly learning from how the system is used. Financial institutions use AI with machine learning models to boost their AML programs and improve regulatory compliance.
However, banks can have problems with AI technology when it is not properly utilized. As a result, it can generate biased outcomes that compromise AML accuracy. Therefore, financial institutions must understand how to use AI correctly to avoid introducing unintended biases into the system. Making sure the AI model does not generate biased predictions is not only ethical. It helps banks preserve their reputation and prevent false reports that could potentially break customers’ trust.
To help banks leverage AI properly, here’s how to use AI technology responsibly with AML programs:
Ensure the Recorded Data Used to Train AI is Correct and Complete
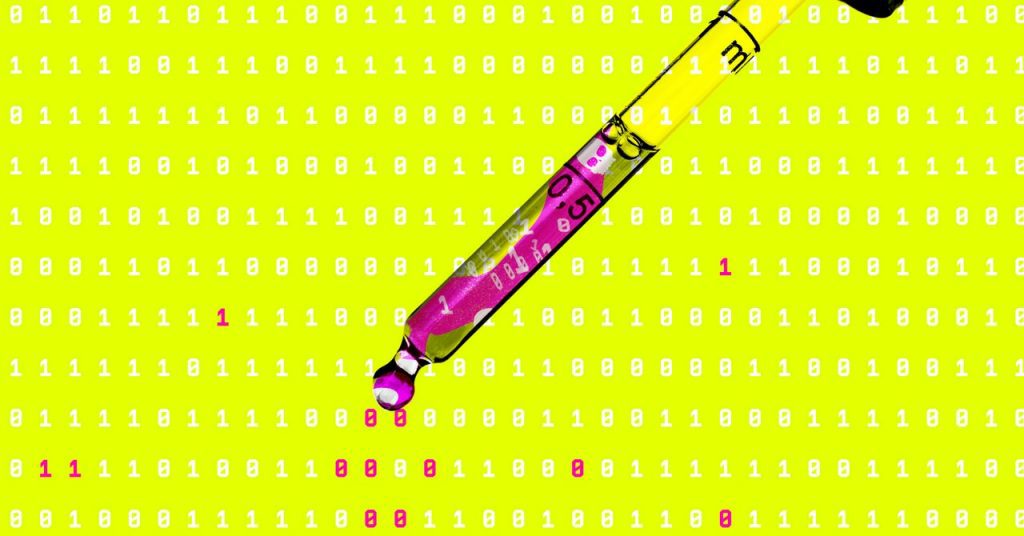
Since AI is highly dependent on data to produce accurate interpretations, AML teams must record correct and complete information. AML teams must carefully review the information and update it, especially if specific details about a client have changed. Banks that established operations before widespread data digitization may take a while to implement this step, but it is necessary. Moreover, AML teams must ensure the data is representative of the clients being monitored. When the initial data is wrong or lacks other representative information, the AI will likely generate biased results.
Be Careful About Inputting Attributes That May Influence Bias
When developing AI machine learning models for AML, the Feature Selection stage can be prone to model feature biases. To address this issue, AML data analytics teams must be cautious of selecting certain information that might impress systematic bias into a model. Information such as net worth, employment data, and even location can influence how AI can interpret outcomes. For instance, seemingly neutral information such as a home address may be taken by IA as placeholder data for other background indicators, such as ethnicity or race. To avoid encoding bias into the system, the AML team must review the kind of attributes that are included in an AI machine learning model.
Compliance Departments Should Communicate Clearly with Data Science Teams
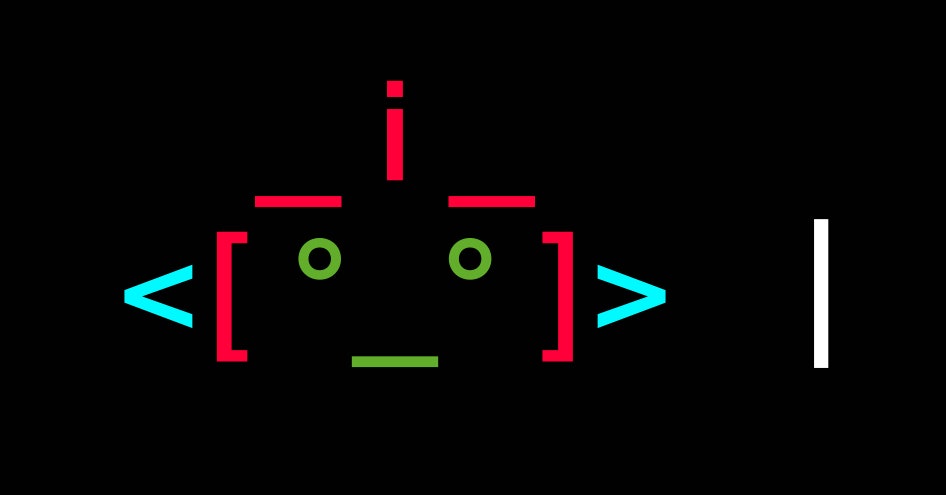
Data scientists and analytics professionals are key stakeholders in developing AI machine learning models for AML systems. This means compliance teams must coordinate with them to build an effective and appropriate AI model for a successful AML program. As such, compliance departments should communicate clear directives with data science teams to stay completely aligned. This includes complying with the bank’s objectives, principles, and guidelines. Clear communication ensures that the AI machine learning model functions according to standards. Chief compliance officers must also encourage data science teams to incorporate bias assessments in the AI model’s performance.
AML Teams Must Focus on Building Interpretable Models
When developing AI models for AML, banks might tend to refer to a black-box model. In the financial industry, a black-box is a program specifically designed to extract certain data that can be used for potential investment plans. Black-box model explanations also vary across program providers, which can create further confusion among compliance departments.
In the case of developing AI models for AML systems, it’s ideal to prioritize building interpretable models over black-box models. This ensures that the system is transparent and can easily be understood. Compliance teams must prioritize providing clear explanations that are contextualized appropriately. Teams should also devise a system that accurately notes whenever someone makes updates to the model. In the long run, this would make it easier to incorporate the AI’s results into regulatory reports, making the data transparent and fully auditable.
Implement Regular AI Model Evaluations to Ensure Consistency
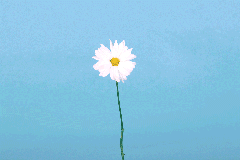
Maintaining AI models for AML systems requires ongoing assessment. It entails regular evaluation and retraining to make sure the AI program steers clear of performance biases. Once an AI model is launched, it must be evaluated by data science teams continuously. This also includes assessing the model’s predictive outcomes for fairness. For instance, changing factors such as new financial products and new customer behavior can influence the AI’s performance. If the team fails to make updates to retrain the system, its performance will decline. Thus, it’s crucial to hold periodic evaluations and retraining to keep the AI model from generating inaccurate and biased results.
Financial institutions have recognized the benefits of AI for strengthening their AML programs. However, banks must understand how to use this technology responsibly to avoid generating biased projections. At the end of the day, AML systems are still reliant on humans–data scientists, analysts, and chief compliance officers–to develop an accurate and efficient AML program.